Extensions
TMT
MSstatsTMT is an R-based package for detecting differentially abundant proteins in shotgun mass spectrometry-based proteomic experiments with tandem mass tag (TMT) labeling. It is applicable to isobaric labeling quantitative proteomics, including iTRAQ and TMT data. MSstatsTMT provides functionalities for two types of analysis: 1) Protein summarization based on peptide quantification data and visualization; 2) Model-based group comparison to detect significant changes in abundance.
PTM
MSstatsPTM provides general statistical methods for quantitative characterization of post-translational modifications (PTMs). Supports DDA, DIA, and tandem mass tag (TMT) labeling. Typically, the analysis involves the quantification of PTM sites (i.e., modified residues) and their corresponding proteins, as well as the integration of the quantification results. MSstatsPTM provides functions for summarization, estimation of PTM site abundance, and detection of changes in PTMs across experimental conditions.
LiP
MSstatsLiP is a tool for LiP peptide and protein significance analysis. Provides functions for summarization, estimation of LiP peptide abundance, and detection of changes across conditions. Utilizes functionality across the MSstats family of packages.
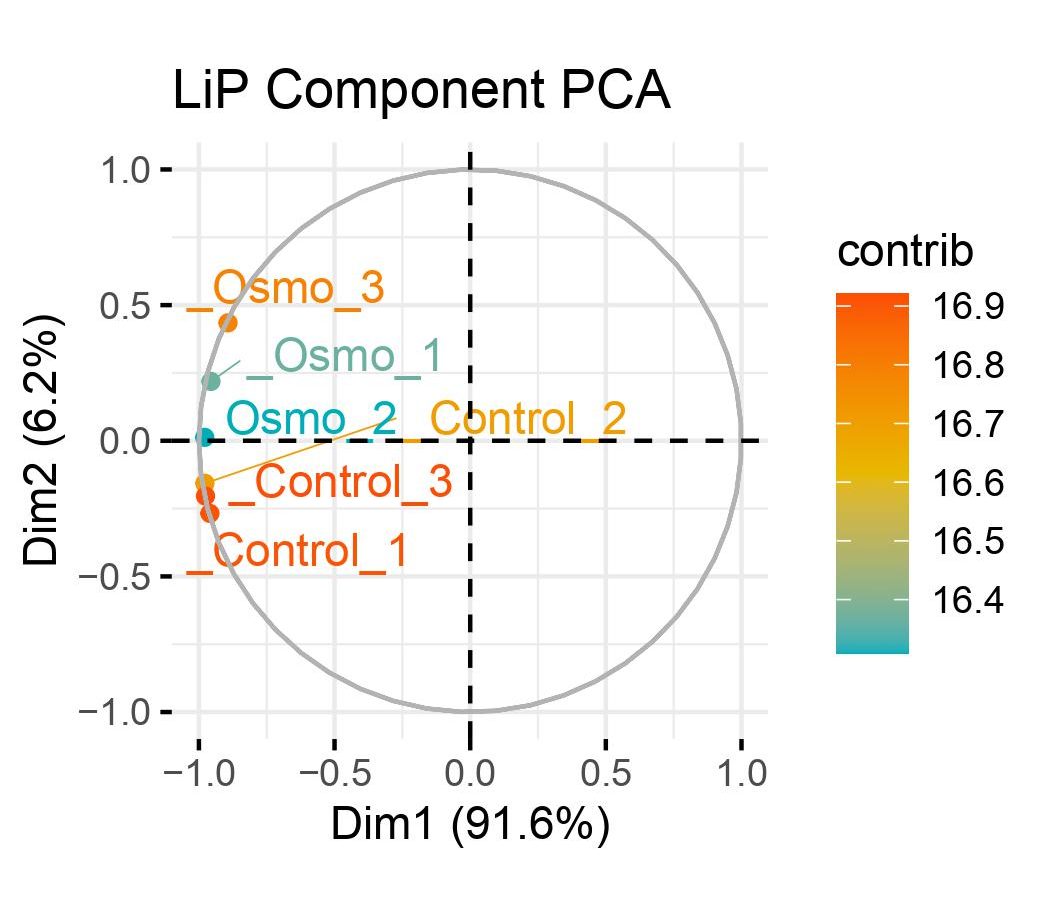
LOB/LOD
MSstatsLOBD performs assay characterization and estimation of limit of blanc (LoB) and limit of detection (LoD). The need for assay characterization is ubiquitous in quantitative mass spectrometry-based proteomics. Although many assay characteristics exist, the limit of blank (LOB) and limit of detection (LOD) are particularly useful figures of merit. LOB and LOD are determined by repeatedly measuring the peak intensities of peptides in samples with known peptide concentrations, and deriving an intensity versus concentration response curve. Most commonly, a weighted linear regression is fit to the intensity-concentration response, and LOB and LOD are estimated from the fit. Linear methods, however, inaccurately characterize assays containing a noise threshold at low concentrations, which is a very common situation. We propose a new approach based on non-linear regression that correctly captures the noise threshold. In absence of a noise threshold, the estimates of LOB/LOD obtained with non-linear statistical modeling are identical to those of weighted linear regression. However, in presence of a noise threshold the non-linear model changed the estimates of LOB/LOD by up to 20-40%. It improved the accuracy of the results, and avoided the unduly optimistic estimation of these figures of merit. We implemented the non-linear regression approach in the open-source R-based software MSstats, and advocate its general use for mass spectrometric protein assay characterization.
QC
MSstatsQC is a longitudinal system suitability monitoring and quality control for proteomic experiments. Statistical process control (SPC) is a general and well-established method of quality control (QC) which can be used to monitor and improve the quality of a process such as LC MS/MS. `MSstatsQC` and `MSstatsQCgui` are two open-source R packages for statistical analysis and monitoring of QC and system suitability testing (SST) samples produced by spectrometry-based proteomic experiments. Our framework termed `MSstatsQC` is available through http://www.msstats.org/msstatsqc. It uses SPC tools to track metrics including total peak area, retention time, full width at half maximum (FWHM) and peak asymmetry for proteomic experiments. We introduce simultaneous and time weighted control charts and change point analysis to monitor mean and variability of metrics. Proposed longitudinal monitoring approach significantly improves the ability of real time monitoring, early detection and prevention of chromatographic and instrumental problems of mass spectrometric assays, thereby, reducing cost of control and failure.
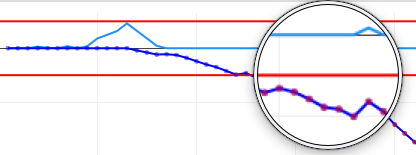